Cómo desplegar NLP: Incrustaciones de texto y búsqueda de vectores
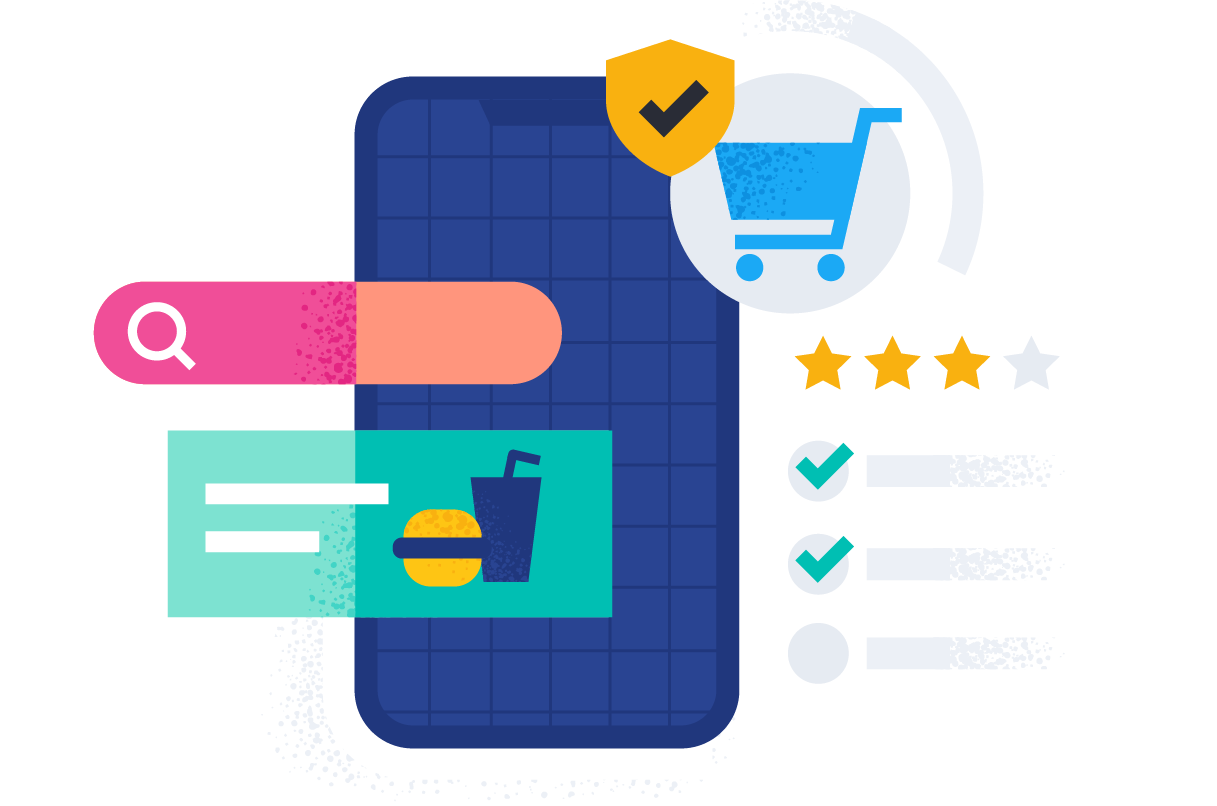
Como parte de nuestra serie de blogs sobre procesamiento del lenguaje natural (NLP), veremos un ejemplo de cómo usar un modelo de incrustación de texto para generar representaciones vectoriales de contenidos textuales y demostrar la búsqueda por similitud de vectores en los vectores generados. Desplegaremos un modelo disponible de forma pública en Elasticsearch y lo usaremos en un pipeline de ingesta a fin de generar incrustaciones a partir de documentos textuales. Luego mostraremos cómo usar esas incrustaciones en la búsqueda por similitud de vectores para encontrar documentos semánticamente similares en una búsqueda dada.
La búsqueda por similitud de vectores o, como se la conoce comúnmente, la búsqueda semántica va más allá de la búsqueda basada en palabras clave tradicional y permite a los usuarios encontrar documentos semánticamente similares que es posible que no tengan palabras clave en común, lo que brinda un rango más amplio de resultados. La búsqueda por similitud de vectores opera con vectores densos y usa la búsqueda k vecino más cercano para encontrar vectores similares. Para ello, los contenidos en formato textual primero deben convertirse a representaciones de vectores numéricos mediante un modelo de incrustación de texto.
Usaremos un set de datos público de la tarea de clasificación de pasajes de MS MARCO para la demostración. Consiste en preguntas reales del motor de búsqueda Microsoft Bing y respuestas reales generadas por seres humanos. Este set de datos es un recurso perfecto para probar la búsqueda por similitud de vectores: en primer lugar, porque el caso de uso de pregunta-respuesta es uno de los más comunes en la búsqueda de vectores y, en segundo lugar, porque las principales publicaciones en la tabla de líderes de MS MARCO usan la búsqueda de vectores de alguna forma.
En nuestro ejemplo, trabajaremos con una muestra de este set de datos, usaremos un modelo para producir incrustaciones de texto y luego ejecutaremos la búsqueda de vectores en él. También esperamos hacer una verificación rápida de la calidad de los resultados producidos a partir de la búsqueda de vectores.
1. Desplegar un modelo de incrustación de texto
El primer paso es instalar un modelo de incrustación de texto. Para nuestro modelo usamos msmarco-MiniLM-L-12-v3 de Hugging Face. Es un modelo de transformación de oraciones que toma una oración o un párrafo y lo mapea a un vector denso de 384 dimensiones. Este modelo está optimizado para la búsqueda semántica y se entrenó específicamente con el set de datos de pasajes de MS MARCO, por lo que es adecuado para nuestra tarea. Además de este modelo, Elasticsearch brinda soporte a varios otros modelos para incrustación de texto. La lista completa está aquí.
Instalamos el modelo con el agente de Docker Eland que compilamos en el ejemplo de NER. Ejecutar un script a continuación importa nuestro modelo al cluster local y lo despliega:
eland_import_hub_model \
--url https://<user>:<password>@localhost:9200/ \
--hub-model-id sentence-transformers/msmarco-MiniLM-L-12-v3 \
--task-type text_embedding \
--start
En esta ocasión, --task-type está configurado en text_embedding y la opción --start se pasa al script Eland para que el modelo se despliegue automáticamente sin tener que iniciarlo en la UI de Model Management. Para acelerar las inferencias, puedes aumentar la cantidad de subprocesos de inferencia con el parámetro inference_threads.
Podemos probar el despliegue exitoso del modelo con este ejemplo en la consola de Kibana:
POST /_ml/trained_models/sentence-transformers__msmarco-minilm-l-12-v3/deployment/_infer
{
"docs": {
"text_field": "how is the weather in jamaica"
}
}
Deberíamos ver el vector denso anticipado como resultado:
{
"predicted_value" : [
0.3345310091972351,
-0.305600643157959,
0.2592800557613373,
…
]
}
2. Cargar datos iniciales
Como mencionamos en la introducción, usamos el set de datos de clasificación de pasajes de MS MARCO. Este set de datos es bastante grande, posee más de 8 millones de pasajes. En nuestro ejemplo, usamos un subconjunto de este que se usó en la etapa de pruebas de 2019 TREC Deep Learning Track. El set de datos msmarco-passagetest2019-top1000.tsv usado para la tarea de reclasificación contiene 200 consultas y, para cada consulta, una lista de pasajes de texto relevantes extraídos mediante un sistema simple de IR. A partir de dicho set de datos, extrajimos todos pasajes únicos con sus ID y los colocamos en un archivo tsv separado, lo que alcanzó un total de 182 469 pasajes. Usamos este archivo como nuestro set de datos.
Usamos la característica de carga de archivos de Kibana para cargar este set de datos. La carga de archivos de Kibana nos permite brindar nombres personalizados para los campos, llamémoslos id con el tipo long, en el caso de las ID de los pasajes, y text con el tipo text, en el caso de los contenidos de los pasajes. El nombre del índice es collection. Luego de la carga, podemos ver un índice llamado collection con 182 469 documentos.
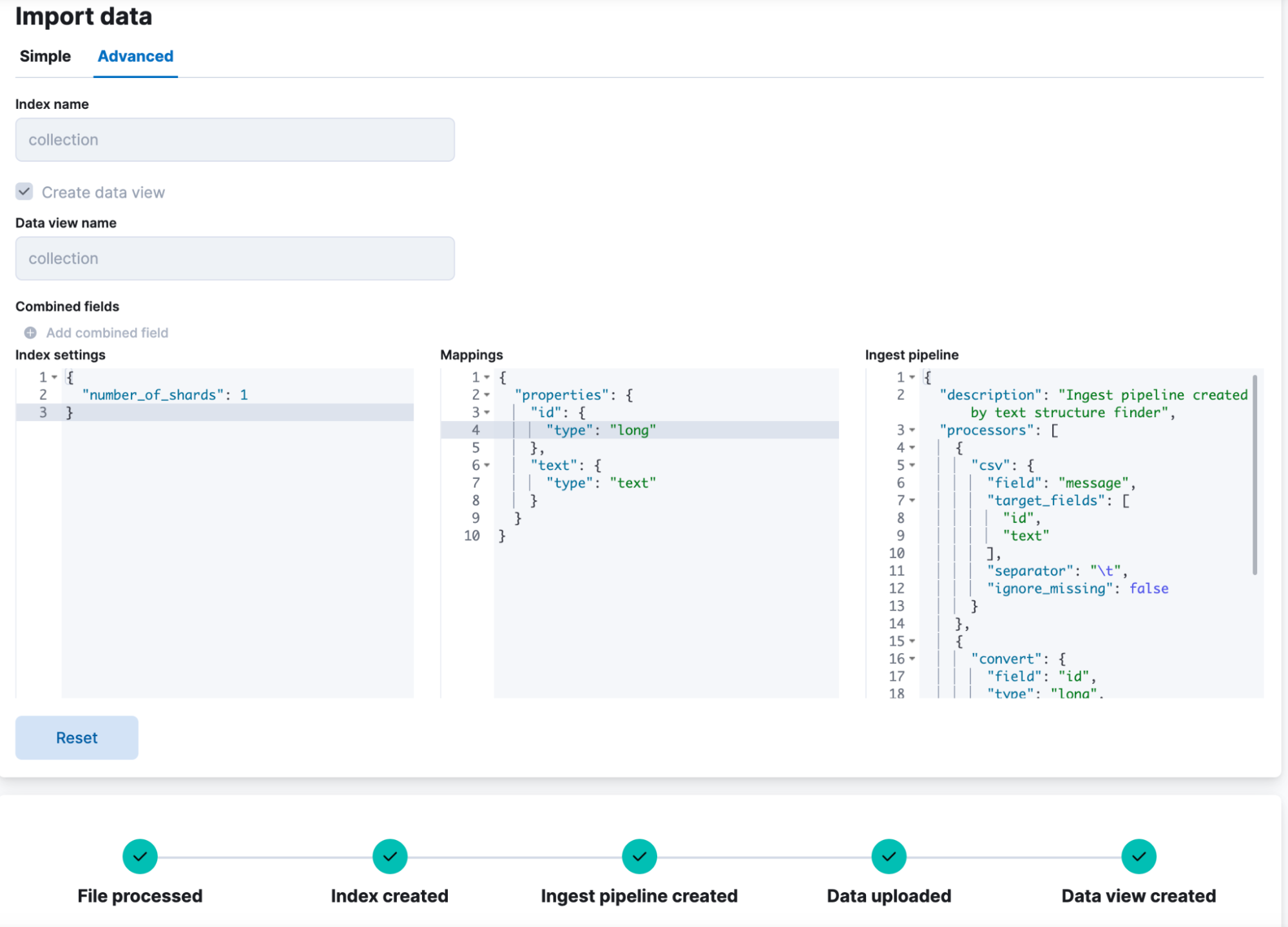
3. Creación del pipeline
Debemos procesar los datos iniciales con un procesador de inferencias que agregará una incrustación para cada pasaje. Para ello, creamos un pipeline de ingesta de incrustación de texto y luego reindexamos los datos iniciales con este pipeline.
En la consola de Kibana, creamos un pipeline de ingesta (como hicimos en el blog anterior), esta vez para las incrustaciones de texto, y lo denominamos text-embeddings. Los pasajes se encuentran en un campo llamado text. Al igual que antes, definiremos un field_map para mapear el texto al campo text_field que espera el modelo. Del mismo modo, el controlador on_failure está configurado para indexar las fallas en un índice diferente:
PUT _ingest/pipeline/text-embeddings
{
"description": "Text embedding pipeline",
"processors": [
{
"inference": {
"model_id": "sentence-transformers__msmarco-minilm-l-12-v3",
"target_field": "text_embedding",
"field_map": {
"text": "text_field"
}
}
}
],
"on_failure": [
{
"set": {
"description": "Index document to 'failed-<index>'",
"field": "_index",
"value": "failed-{{{_index}}}"
}
},
{
"set": {
"description": "Set error message",
"field": "ingest.failure",
"value": "{{_ingest.on_failure_message}}"
}
}
]
}
4. Reindexar los datos
Debemos reindexar los documentos del índice collection en el nuevo índice collection-with-embeddings haciendo pasar los documentos por el pipeline text-embeddings, de modo que los documentos en el índice collection-with-embeddings tengan un campo adicional para las incrustaciones de los pasajes. Pero antes de hacerlo, debemos crear y definir un mapeo para nuestro índice de destino, en especial para el campo text_embedding.predicted_value en el que el procesador de ingesta almacenará las incrustaciones. Si no lo hacemos, las incrustaciones se indexarán en campos float regulares y no se podrán usar para la búsqueda por similitud de vectores. El modelo que usamos produce incrustaciones como vectores de 384 dimensiones, por lo tanto usamos el tipo de campo dense_vector indexado con 384 dimensiones, de esta manera:
PUT collection-with-embeddings
{
"mappings": {
"properties": {
"text_embedding.predicted_value": {
"type": "dense_vector",
"dims": 384,
"index": true,
"similarity": "cosine"
},
"text": {
"type": "text"
}
}
}
}
Por último, estamos listos para reindexar. Dado que la reindexación tomará un tiempo para procesar todos los documentos y realizar inferencias con base en ellos, realizamos la indexación en segundo plano invocando la API con el marcador wait_for_completion=false.
POST _reindex?wait_for_completion=false
{
"source": {
"index": "collection"
},
"dest": {
"index": "collection-with-embeddings",
"pipeline": "text-embeddings"
}
}
Lo anterior devuelve una ID de tarea. Podemos monitorear el progreso de la tarea con lo siguiente:
GET _tasks/<task_id>
Como alternativa, rastrea el progreso observando el aumento del recuento de inferencias en la API de estadísticas de modelo o la UI de estadísticas de modelo.

Los documentos reindexados ahora contienen los resultados de las inferencias: las incrustaciones de vectores. A modo de ejemplo, uno de los documentos se ve así:
{
"id": "G7PPtn8BjSkJO8zzChzT",
"text": "This is the definition of RNA along with examples of types of RNA molecules. This is the definition of RNA along with examples of types of RNA molecules. RNA Definition",
"text_embedding":
{
"predicted_value":
[
0.057356324046850204,
0.1602816879749298,
-0.18122544884681702,
0.022277727723121643,
....
],
"model_id": "sentence-transformers__msmarco-minilm-l-12-v3"
}
}
5. Búsqueda por similitud de vectores
Actualmente no brindamos soporte para la generación implícita de incrustaciones a partir de los términos de búsqueda durante una solicitud de búsqueda, por lo que nuestra búsqueda semántica se organiza como un proceso de 2 pasos:
- Obtener una incrustación de texto a partir de una búsqueda textual. Para ello, usamos la API _infer de nuestro modelo.
- Usar la búsqueda de vectores para encontrar documentos semánticamente similares al texto de búsqueda. En Elasticsearch v8.0, presentamos un nuevo endpoint _knn_search que permite la búsqueda eficiente de vecinos más cercanos aproximados en los campos dense_vector indexados. Usamos la API _knn_search para encontrar los documentos más cercanos.
Por ejemplo, dada una búsqueda textual "how is the weather in jamaica", primero ejecutamos la API _infer para obtener su incrustación como vector denso:
POST /_ml/trained_models/sentence-transformers__msmarco-minilm-l-12-v3/deployment/_infer
{
"docs": {
"text_field": "how is the weather in jamaica"
}
}
Luego, conectamos el vector denso resultante a _knn_search de la siguiente manera:
GET collection-with-embeddings/_knn_search
{
"knn": {
"field": "text_embedding.predicted_value",
"query_vector": [
0.3345310091972351,
-0.305600643157959,
0.2592800557613373,
…
],
"k": 10,
"num_candidates": 100
},
"_source": [
"id",
"text"
]
}
Como resultado, obtenemos los 10 resultados principales más cercanos a los documentos de búsqueda ordenados según su proximidad a la búsqueda:
"hits" : [
{
"_index" : "collection-with-embeddings",
"_id" : "47TPtn8BjSkJO8zzKq_o",
"_score" : 0.94591534,
"_source" : {
"id" : 434125,
"text" : "The climate in Jamaica is tropical and humid with warm to hot temperatures all year round. The average temperature in Jamaica is between 80 and 90 degrees Fahrenheit. Jamaican nights are considerably cooler than the days, and the mountain areas are cooler than the lower land throughout the year. Continue Reading."
}
},
{
"_index" : "collection-with-embeddings",
"_id" : "3LTPtn8BjSkJO8zzKJO1",
"_score" : 0.94536424,
"_source" : {
"id" : 4498474,
"text" : "The climate in Jamaica is tropical and humid with warm to hot temperatures all year round. The average temperature in Jamaica is between 80 and 90 degrees Fahrenheit. Jamaican nights are considerably cooler than the days, and the mountain areas are cooler than the lower land throughout the year"
}
},
{
"_index" : "collection-with-embeddings",
"_id" : "KrXPtn8BjSkJO8zzPbDW",
"_score" : 0.9432083,
"_source" : {
"id" : 190804,
"text" : "Quick Answer. The climate in Jamaica is tropical and humid with warm to hot temperatures all year round. The average temperature in Jamaica is between 80 and 90 degrees Fahrenheit. Jamaican nights are considerably cooler than the days, and the mountain areas are cooler than the lower land throughout the year. Continue Reading"
}
},
...
6. Verificación rápida
Como usamos solo un subconjunto del set de datos de MS MARCO, no podemos hacer una evaluación completa. En cambio, lo que podemos hacer es una verificación simple en algunas búsquedas para entender si en realidad obtenemos resultados relevantes y no aleatorios. De las valoraciones de TREC 2019 Deep Learning Track correspondientes a la tarea de clasificación de pasajes, tomamos las 3 últimas búsquedas, las enviamos a nuestra búsqueda por similitud de vectores, obtenemos los 10 resultados principales y consultamos las valoraciones de TREC para conocer la relevancia de los resultados que recibimos. En la tarea de clasificación de pasajes, los pasajes se evalúan según una escala de cuatro puntos Irrelevante (0), Relacionado (el pasaje es sobre el tema, pero no responde la pregunta) (1), Muy relevante (2) y Perfectamente relevante (3).
Ten en cuenta que nuestra verificación no es una evaluación rigurosa, se usa solo para la demostración rápida. Como solo indexamos pasajes que se sabe que están relacionados con las búsquedas, es una tarea mucho más sencilla que la tarea de recuperación de pasajes original. Nuestro objetivo para el futuro es hacer una evaluación rigurosa del set de datos de MS MARCO.
La búsqueda 1124210 "tracheids are part of _____" enviada a nuestra búsqueda de vectores devuelve los resultados siguientes:
ID de pasaje | Clasificación de relevancia | Pasaje |
---|---|---|
2258591 | 2 - muy relevante | Tracheid of oak shows pits along the walls. It is longer than a vessel element and has no perforation plates. Tracheids are elongated cells in the xylem of vascular plants that serve in the transport of water and mineral salts.Tracheids are one of two types of tracheary elements, vessel elements being the other. Tracheids, unlike vessel elements, do not have perforation plates.racheids provide most of the structural support in softwoods, where they are the major cell type. Because tracheids have a much higher surface to volume ratio compared to vessel elements, they serve to hold water against gravity (by adhesion) when transpiration is not occurring. |
2258592 | 3 - perfectamente relevante | Tracheid. a dead lignified plant cell that functions in water conduction. Tracheids are found in the xylem of all higher plants except certain angiosperms, such as cereals and sedges, in which the water-conducting function is performed by vessels, or tracheae.Tracheids are usually polygonal in cross section; their walls have annular, spiral, or scalene thickenings or rimmed pores.racheids are found in the xylem of all higher plants except certain angiosperms, such as cereals and sedges, in which the water-conducting function is performed by vessels, or tracheae. Tracheids are usually polygonal in cross section; their walls have annular, spiral, or scalene thickenings or rimmed pores. |
2258596 | 2 - muy relevante | Woody angiosperms have also vessels. The mature tracheids form a column of superposed, cylindrical dead cells whose end walls have been perforated, resulting in a continuous tube called vessel (trachea). Tracheids are found in all vascular plants and are the only conducting elements in gymnosperms and ferns. Tracheids have Pits on their end walls. Pits are not nearly as efficient for water translocation as Perforation Plates found in vessel elements. Woody angiosperms have also vessels. The mature tracheids form a column of superposed, cylindrical dead cells whose end walls have been perforated, resulting in a continuous tube called vessel (trachea). Tracheids are found in all vascular plants and are the only conducting elements in gymnosperms and ferns |
2258595 | 2 - muy relevante | Summary: Vessels have perforations at the end plates while tracheids do not have end plates. Tracheids are derived from single individual cells while vessels are derived from a pile of cells. Tracheids are present in all vascular plants whereas vessels are confined to angiosperms. Tracheids are thin whereas vessel elements are wide. Tracheids have a much higher surface-to-volume ratio as compared to vessel elements. Vessels are broader than tracheids with which they are associated. Morphology of the perforation plate is different from that in tracheids. Tracheids are thin whereas vessel elements are wide. Tracheids have a much higher surface-to-volume ratio as compared to vessel elements. Vessels are broader than tracheids with which they are associated. Morphology of the perforation plate is different from that in tracheids. |
131190 | 3 - perfectamente relevante | Xylem tracheids are pointed, elongated xylem cells, the simplest of which have continuous primary cell walls and lignified secondary wall thickenings in the form of rings, hoops, or reticulate networks. |
7443586 | 2 - muy relevante | 1 The xylem tracheary elements consist of cells known as tracheids and vessel members, both of which are typically narrow, hollow, and elongated. Tracheids are less specialized than the vessel members and are the only type of water-conducting cells in most gymnosperms and seedless vascular plants. |
181177 | 2 - muy relevante | In most plants, pitted tracheids function as the primary transport cells. The other type of tracheary element, besides the tracheid, is the vessel element. Vessel elements are joined by perforations into vessels. In vessels, water travels by bulk flow, as in a pipe, rather than by diffusion through cell membranes. |
2947055 | 0 - irrelevante | Cholesterol belongs to the groups of lipids called _______.holesterol belongs to the groups of lipids called _______. |
6541866 | 2 - muy relevante | In most plants, pitted tracheids function as the primary transport cells. The other type of tracheary element, besides the tracheid, is the vessel element. Vessel elements are joined by perforations into vessels. In vessels, water travels by bulk flow, as in a pipe, rather than by diffusion through cell membranes. In most plants, pitted tracheids function as the primary transport cells. The other type of tracheary element, besides the tracheid, is the vessel element. Vessel elements are joined by perforations into vessels. In vessels, water travels by bulk flow, as in a pipe, rather than by diffusion through cell membranes. |
La búsqueda 1129237 "hydrogen is a liquid below what temperature" devuelve los siguientes resultados:
ID de pasaje | Clasificación de relevancia | Pasaje |
---|---|---|
8588222 | 0 - irrelevante | Answer to: Hydrogen is a liquid below what temperature? By signing up, you'll get thousands of step-by-step solutions to your homework questions.... for Teachers for Schools for Companies |
128984 | 3 - perfectamente relevante | Hydrogen gas has the molecular formula H 2. At room temperature and under standard pressure conditions, hydrogen is a gas that is tasteless, odorless and colorless. Hydrogen can exist as a liquid under high pressure and an extremely low temperature of 20.28 kelvin (−252.87°C, −423.17 °F). Hydrogen is often stored in this way as liquid hydrogen takes up less space than hydrogen in its normal gas form. Liquid hydrogen is also used as a rocket fuel. |
8588219 | 3 - perfectamente relevante | User: Hydrogen is a liquid below what temperature? a. 100 degrees C c. -183 degrees C b. -253 degrees C d. 0 degrees C Weegy: Hydrogen is a liquid below 253 degrees C. User: What is the boiling point of oxygen? a. 100 degrees C c. -57 degrees C b. 8 degrees C d. -183 degrees C Weegy: The boiling point of oxygen is -183 degrees C. |
3905057 | 3 - perfectamente relevante | Hydrogen is a colorless, odorless, tasteless gas. Its density is the lowest of any chemical element, 0.08999 grams per liter. By comparison, a liter of air weighs 1.29 grams, 14 times as much as a liter of hydrogen. Hydrogen changes from a gas to a liquid at a temperature of -252.77°C (-422.99°F) and from a liquid to a solid at a temperature of -259.2°C (-434.6°F). It is slightly soluble in water, alcohol, and a few other common liquids. |
4254811 | 3 - perfectamente relevante | At STP (standard temperature and pressure) hydrogen is a gas. It cools to a liquid at -423 °F, which is only about 37 degrees above absolute zero. Eleven degrees cooler, at … -434 °F, it starts to solidify. |
2697752 | 2 - muy relevante | Hydrogen's state of matter is gas at standard conditions of temperature and pressure. Hydrogen condenses into a liquid or freezes solid at extremely cold... Hydrogen's state of matter is gas at standard conditions of temperature and pressure. Hydrogen condenses into a liquid or freezes solid at extremely cold temperatures. Hydrogen's state of matter can change when the temperature changes, becoming a liquid at temperatures between minus 423.18 and minus 434.49 degrees Fahrenheit. It becomes a solid at temperatures below minus 434.49 F.Due to its high flammability, hydrogen gas is commonly used in combustion reactions, such as in rocket and automobile fuels. |
6080460 | 3 - perfectamente relevante | Hydrogen can exist as a liquid under high pressure and an extremely low temperature of 20.28 kelvin (−252.87°C, −423.17 °F). Hydrogen is often stored in this way as liquid hydrogen takes up less space than hydrogen in its normal gas form. Liquid hydrogen is also used as a rocket fuel. Hydrogen is found in large amounts in giant gas planets and stars, it plays a key role in powering stars through fusion reactions. Hydrogen is one of two important elements found in water (H 2 O). Each molecule of water is made up of two hydrogen atoms bonded to one oxygen atom. |
128989 | 3 - perfectamente relevante | Confidence votes 11.4K. At STP (standard temperature and pressure) hydrogen is a gas. It cools to a liquid at -423 °F, which is only about 37 degrees above absolute zero. Eleven degrees cooler, at -434 °F, it starts to solidify. |
1959030 | 0 - irrelevante | While below 4 °C the breakage of hydrogen bonds due to heating allows water molecules to pack closer despite the increase in the thermal motion (which tends to expand a liquid), above 4 °C water expands as the temperature increases. Water near the boiling point is about 4% less dense than water at 4 °C (39 °F) |
3905800 | 0 - irrelevante | Hydrogen is the lightest of the elements with an atomic weight of 1.0. Liquid hydrogen has a density of 0.07 grams per cubic centimeter, whereas water has a density of 1.0 g/cc and gasoline about 0.75 g/cc. These facts give hydrogen both advantages and disadvantages. |
La búsqueda 1133167 "how is the weather in jamaica" devuelve los siguientes resultados:
ID de pasaje | Clasificación de relevancia | Pasaje |
---|---|---|
434125 | 3 - perfectamente relevante | The climate in Jamaica is tropical and humid with warm to hot temperatures all year round. The average temperature in Jamaica is between 80 and 90 degrees Fahrenheit. Jamaican nights are considerably cooler than the days, and the mountain areas are cooler than the lower land throughout the year. |
4498474 | 3 - perfectamente relevante | The climate in Jamaica is tropical and humid with warm to hot temperatures all year round. The average temperature in Jamaica is between 80 and 90 degrees Fahrenheit. Jamaican nights are considerably cooler than the days, and the mountain areas are cooler than the lower land throughout the year. |
190804 | 3 - perfectamente relevante | Quick Answer. The climate in Jamaica is tropical and humid with warm to hot temperatures all year round. The average temperature in Jamaica is between 80 and 90 degrees Fahrenheit. Jamaican nights are considerably cooler than the days, and the mountain areas are cooler than the lower land throughout the year. Continue Reading. |
1824479 | 3 - perfectamente relevante | A: The climate in Jamaica is tropical and humid with warm to hot temperatures all year round. The average temperature in Jamaica is between 80 and 90 degrees Fahrenheit. Jamaican nights are considerably cooler than the days, and the mountain areas are cooler than the lower land throughout the year. |
1824480 | 3 - perfectamente relevante | Quick Answer. The climate in Jamaica is tropical and humid with warm to hot temperatures all year round. The average temperature in Jamaica is between 80 and 90 degrees Fahrenheit. Jamaican nights are considerably cooler than the days, and the mountain areas are cooler than the lower land throughout the year. |
1824488 | 2 - muy relevante | Learn About the Weather of Jamaica The weather patterns you'll encounter in Jamaica can vary dramatically around the island Regardless of when you visit, the tropical climate and warm temperatures of Jamaica essentially guarantee beautiful weather during your vacation. Average temperatures in Jamaica range between 80 degrees Fahrenheit and 90 degrees Fahrenheit, with July and August being the hottest months and February the coolest. |
4922619 | 2 - muy relevante | Weather. Jamaica averages about 80 degrees year-round, so climate is less a factor in booking travel than other destinations. The days are warm and the nights are cool. Rain usually falls for short periods in the late afternoon, with sunshine the rest of the day. |
190806 | 2 - muy relevante | Siempre es importante saber cómo será el clima en Jamaica antes de planificar las vacaciones. For the most part, the average temperature in Jamaica is between 80 °F and 90 °F (27 °FCelsius-29 °Celsius). Luckily, the weather in Jamaica is always vacation friendly. You will hardly experience long periods of rain fall, and you will become accustomed to weeks upon weeks of sunny weather. |
2613296 | 2 - muy relevante | Average temperatures in Jamaica range between 80 degrees Fahrenheit and 90 degrees Fahrenheit, with July and August being the hottest months and February the coolest. Temperatures in Jamaica generally vary approximately 10 degrees from summer to winter |
1824486 | 2 - muy relevante | The climate in Jamaica is tropical and humid with warm to hot temperatures all year round. The average temperature in Jamaica is between 80 and 90 degrees Fahrenheit. Jamaican nights are considerably... |
Como podemos ver, para las tres búsquedas Elasticsearch devolvió en su mayoría resultados relevantes, y los resultados principales de todas las búsquedas fueron mayormente muy o perfectamente relevantes.
Prueba
El NLP es una característica poderosa en el Elastic Stack con un roadmap emocionante. Descubre características nuevas y mantente al tanto de los desarrollos más recientes creando tu cluster en Elastic Cloud. Suscríbete para una prueba gratuita de 14 días hoy y prueba los ejemplos de este blog.
Si deseas conocer más sobre NLP, lee:
- How to deploy NLP: Named entity recognition (NER) example (Cómo desplegar NLP: Ejemplo de reconocimiento de entidades con nombre [NER])
- How to deploy NLP: Sentiment Analysis Example (Cómo desplegar NLP: Ejemplo de análisis de sentimiento)
- How to deploy natural language processing (NLP): Getting started (Cómo desplegar el procesamiento de lenguaje natural [NLP]: Primeros pasos)