The future of generative AI in public sector
Takeaways from the Elastic and IDC virtual event
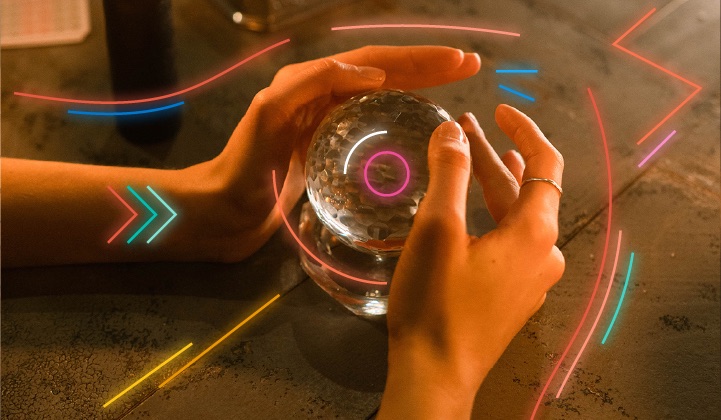
Recently, I sat down with Adelaide O’Brien, research vice president at IDC Government Insights, to discuss the current and future state of generative AI in the public sector worldwide. The full conversation is available to view on demand, but I also wanted to highlight some of the takeaways from the discussion. Our goal was to discuss use cases we’re seeing right now, what roadblocks public organizations are facing, and trade best practices on how organizations can harness the value of generative AI for employees, constituents, and larger digital transformation.
Generative AI in public sector: The current state
IDC research shows that 59% of government agencies are in the beginning stages of generative AI usage at their organizations (compared with 16% that are “investing significantly.”) Looking forward, IDC shared additional insights on how public sector leaders see their near-term generative AI roadmap:
62% of governments around the world say they would use AI in customer service and support in the next 12 months.
49% of educational institutions globally indicate that conversational apps (e.g., chatbots and voice bots) have the most promise for near-term use.
Going a step further, IDC outlines the progression of how public sector organizations will likely adopt and integrate generative AI over the next few years, based on their research as well as advisory conversations with government clients.
The 3 horizons of generative AI use cases in public sector
IDC lays out government use cases in three stages, or horizons, based on organizational maturity level. Unlike some private sector counterparts that have their eyes on revenue potential of generative AI integration, public sector organizations, so far, are proceeding with a more cautious, crawl-walk-run approach.
Horizon one: The first horizon, according to IDC, is incremental innovation happening over the next year or so, as organizations test the waters of generative AI, primarily internally. Initially, use cases are focused on employee productivity and satisfaction, such as pilot programs around internal contract management, procurement, and code creation via sandbox environments. In other words, taking complex, repetitive data-centric tasks and simplifying them through generative AI applications in combination with an agency’s proprietary data. Once these pilot programs get off the ground, organizations plan to expand to use cases that affect external stakeholders, such as improving a constituent's help desk or call center experience by connecting them with personalized, relevant data.
Horizon two: Once an organization feels fairly comfortable with the generative AI culture shift occurring in the first horizon, use cases can expand into more disruptive innovation. This horizon is one that IDC expects to be prevalent within the next few years. The use cases here connect “the front office to the back office” and take advantage of intelligent automation. Examples include critical infrastructure protection, cross-agency data sharing for investigations, and benefits fraud protection.
Horizon three: Use cases in the third and final horizon of IDC’s framework continue to expand in scope and include new business models and integration across complex ecosystems. Here, organizations are planning on a holistic level around systemic topics such as digital legislation, national intelligence superiority, and smart, connected campuses.
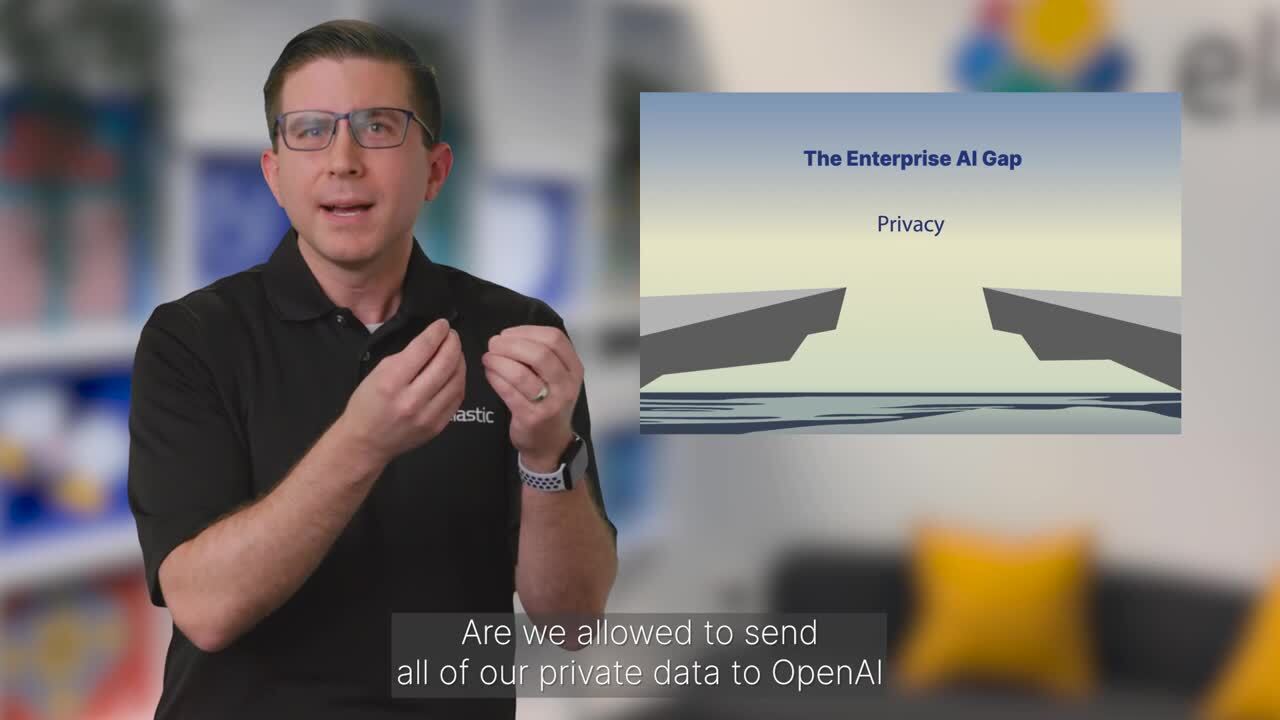
Generative AI at scale requires security and trust
As promising as the horizons of generative AI are, leaders are also facing concerns around data privacy, employee satisfaction, and ethics and compliance. According to IDC data, 43% of global government leaders are concerned that generative AI jeopardizes their control of data and intellectual property, and 41% are worried that generative AI use will expose them to brand and regulatory risks.
For public sector, security and trust are essential for any generative AI implementation — trust in private sector partners, trust in policies and ethical guidelines, and trust that private data remains private. As Adelaide O’Brien noted, “Government will only be able to deliver gen AI value at scale by centering trust.” For public sector organizations, this means strategically thinking through policies and guide rails for responsible AI, including:
Building an AI roadmap for your entire organization
Designing an intelligent architecture
Mapping the skills required for implementation and success
Ensuring your sensitive data isn’t training the large language model (LLM)
Keeping data on sovereign soil
Making sure you own your encryption keys
Critical in all of the above considerations is the “human-in-the-loop” approach, which ensures that generative AI output is cross-checked by humans for misinformation, especially given the potential for generative AI hallucinations.
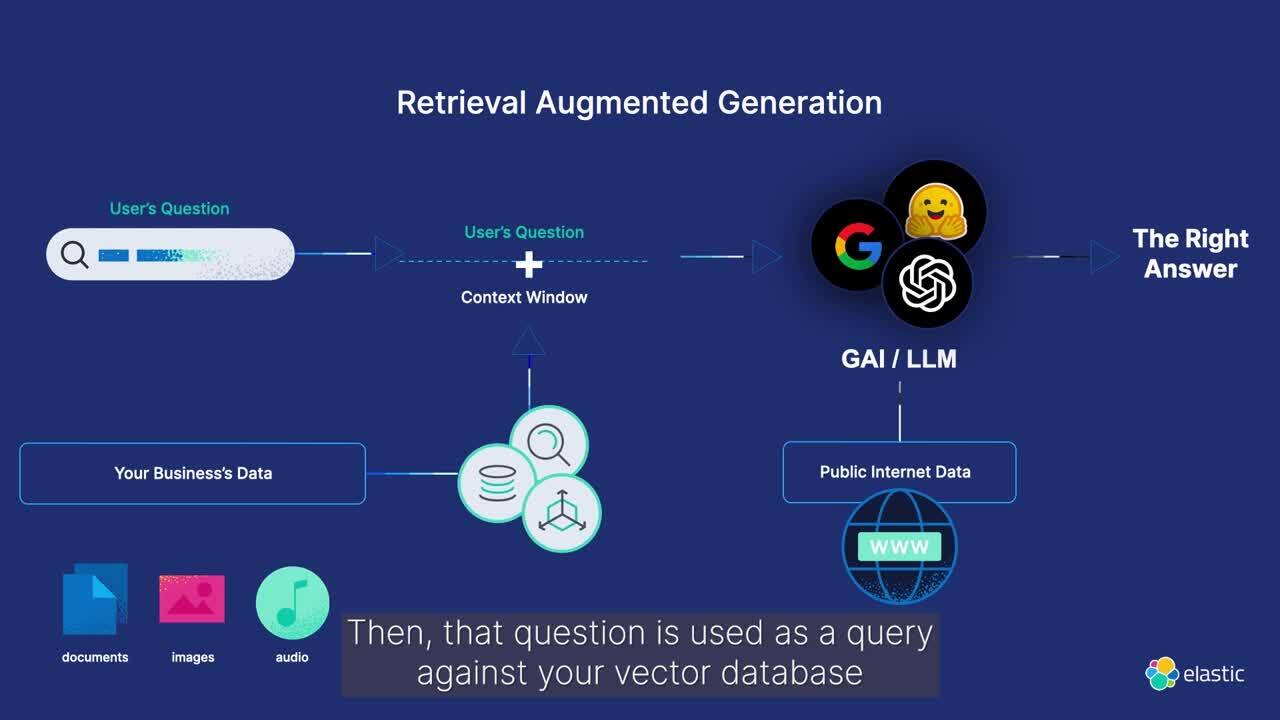
Using retrieval augmented generation to ground models
IDC notes that 36% of government leaders globally have concerns about accuracy or potential toxicity (bias, hallucinations in the outputs) of generative AI use. To make sure that generative AI output is as accurate and timely as possible, both IDC and Elastic® recommend using retrieval augmented generation (RAG). RAG is a natural language processing technique that enables organizations to use their own proprietary data with generative AI in order to improve the quality of the content output. By leveraging your own domain-specific data, RAG grounds LLMs by providing relevant internal context to the generative AI search query, which increases accuracy and reduces hallucinations.
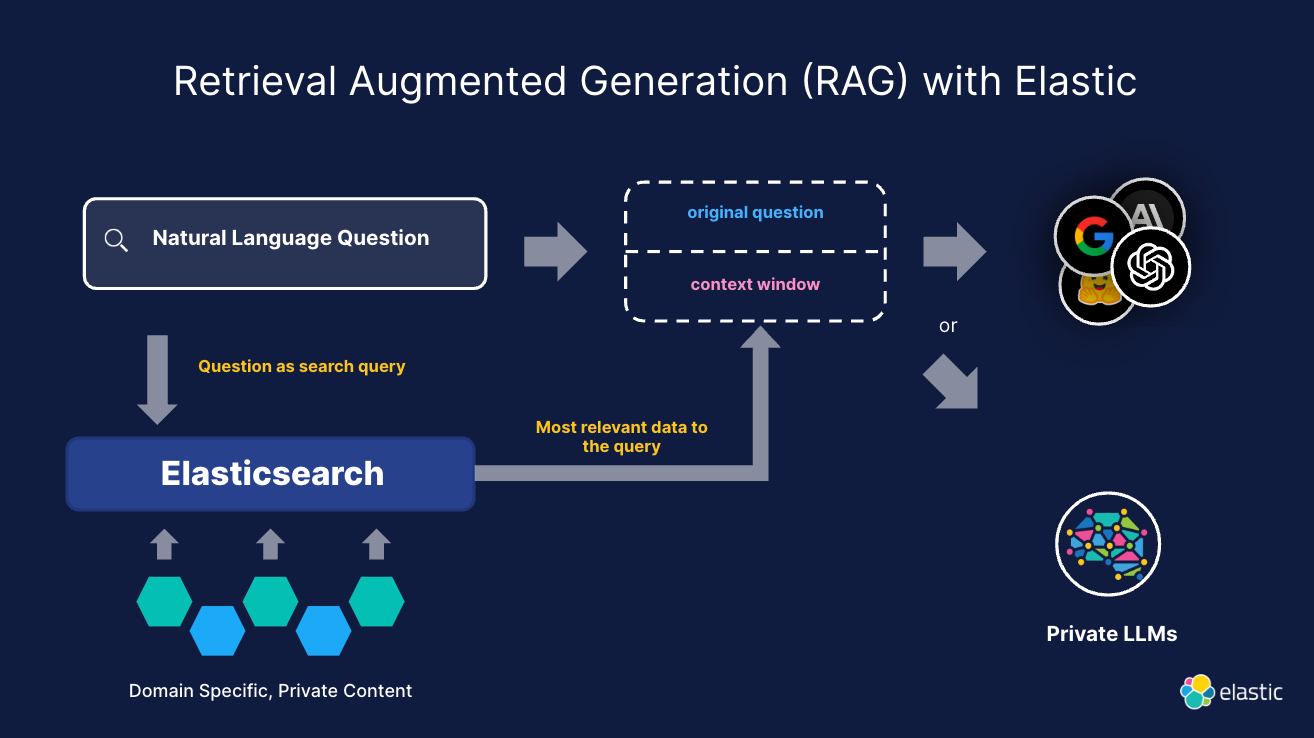
How RAG with Elastic can benefit public sector
Grounded on facts: Use synced data in Elastic for accurate, up-to-date mission-specific results that pass via context window to generative AI models.
Flexibility for superior relevance: Bring your own transformer models into Elastic, integrate with third-party models, or use Elastic’s Learned Sparse EncodeR (ELSER).
Privacy and security: Apply Elastic’s native support for role-based access control for chat and question-answering apps.
Cost-effective: Use smaller LLMs, reducing inferences costs by two orders of magnitude compared to fine-tuning or relying on LLM-based knowledge.
Listen to the full virtual fireside chat
The release and timing of any features or functionality described in this post remain at Elastic's sole discretion. Any features or functionality not currently available may not be delivered on time or at all.
In this blog post, we may have used or referred to third party generative AI tools, which are owned and operated by their respective owners. Elastic does not have any control over the third party tools and we have no responsibility or liability for their content, operation or use, nor for any loss or damage that may arise from your use of such tools. Please exercise caution when using AI tools with personal, sensitive or confidential information. Any data you submit may be used for AI training or other purposes. There is no guarantee that information you provide will be kept secure or confidential. You should familiarize yourself with the privacy practices and terms of use of any generative AI tools prior to use.
Elastic, Elasticsearch, ESRE, Elasticsearch Relevance Engine and associated marks are trademarks, logos or registered trademarks of Elasticsearch N.V. in the United States and other countries. All other company and product names are trademarks, logos or registered trademarks of their respective owners.