AI for the public good: The future is bright
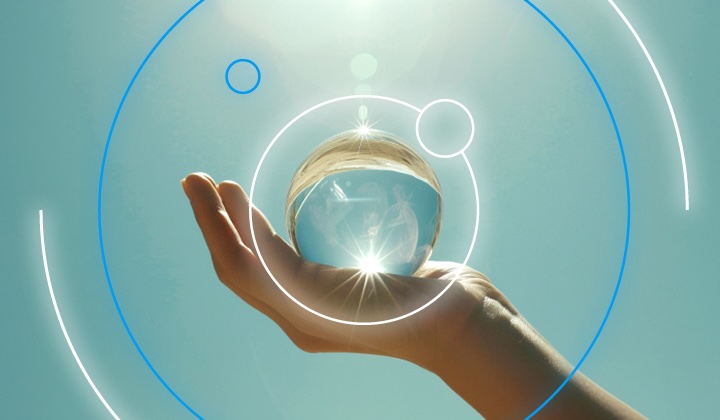
How do we measure the impact of AI in government? Without a doubt, the benefits that AI will bring to operational processes and decision-making have been discussed at great length — from automating workflows to saving costs to reducing duplicated efforts.
But for organisations whose goals centre on serving the public, these benefits of AI aren’t limited to business indicators, such as efficiency or revenue growth. Rather, the potential efficiencies and cost-savings from AI can open up space and resources to devote to delivering meaningful public services that improve the quality of life for people around the globe. It may sound simplistic and idealistic — but it’s already happening.
In the UK, for example, the government has assembled an AI-focused team rapidly developing pilot programs to improve services that directly impact taxpayers and citizens. Initiatives include reducing fraud and error in pharmacies, efficiently moving asylum claimants from hotels, and building a tool to summarize responses to government consultations. These programs have the potential to save taxpayers billions of dollars in addition to improving the overall health and well-being of UK citizens.
In the US, the Government Accountability Office (GAO) estimates that there are over 1,200 AI use cases either underway or planned among federal government agencies — from improving customer support to enhancing benefits programs to analyzing weather hazards. In the UK, 74 AI use cases are in process among government bodies.
When you automate, there’s more room for impact
With public sector organisations typically more strained for resources and personnel, AI can offer significant opportunities to automate tedious tasks and free up time for staff to focus on more strategic, impactful initiatives. Regional research is backing up this idea with the following estimations:
In the UK, it’s estimated that AI can easily automate 84% of citizen-facing transactions and has the potential to save £200 billion each year.
- In the US, it’s predicted that the public sector will see $519 billion in productivity gains from generative AI by 2033.
- In Australia, generative AI has the potential to add between $45-$115 billion each year to the Australian economy, primarily through enhanced productivity.
Responsible and ethical AI at the forefront
A recent Elastic study on global generative AI implementation found that 99% of respondents recognize the positive impacts generative AI can have on their organization. At the same time, 89% of respondents report that their use of generative AI is being slowed. In the UK, only 37% of government bodies surveyed by the National Audit Office (NAO) were actively using AI.
Factors slowing generative AI implementation globally
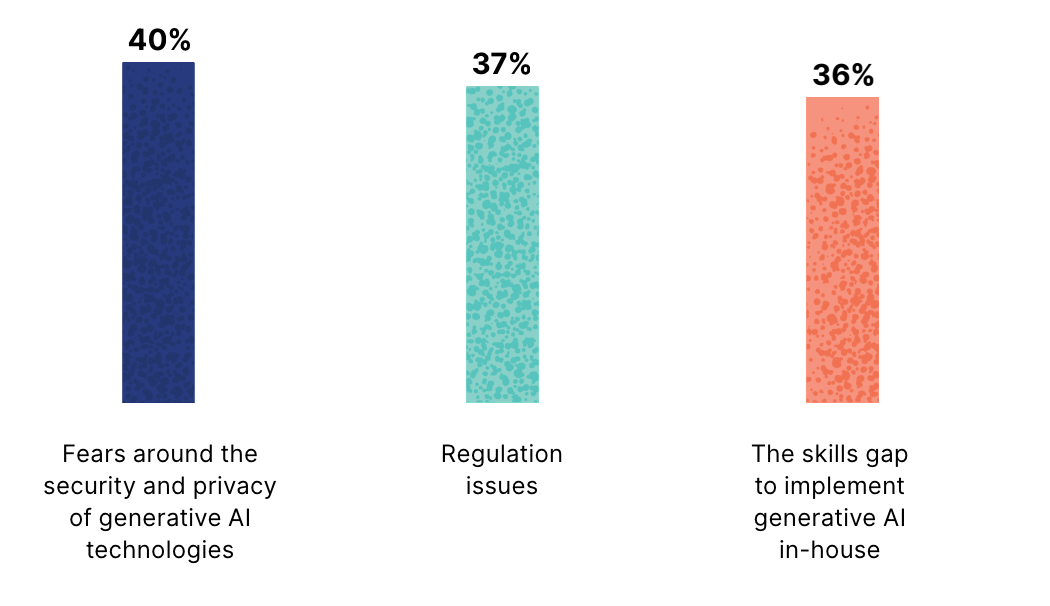
As the graph above shows, the most common factor impeding fast AI implementation is the concern around privacy and security. Public sector officials have a responsibility to guarantee that sensitive data remains protected and that their teams are not feeding their proprietary data directly into a publicly available generative AI application. Government officials and other public sector leaders have understably been hesitant to implement generative AI without assurances of data privacy and responsible use.
In addition to security and privacy concerns, lack of modern data and IT strategies are slowing AI adoption in the public sector. The NAO report out of the UK found that “limited access to good-quality data was a barrier to implementing AI and central government support was important to address this.” Building a strong data foundation and modernising legacy systems will take time and concerted action, which further challenges AI adoption.
Overcoming AI implementation barriers
To speed up AI adoption and help citizens see the positive effects of AI sooner, there are a few tangible steps agencies can take.
While government agencies are understandably daunted at the prospect of reconfiguring their underlying data strategy, there are technology platforms that enable organisations to get more utility and insights out of the data they already have — no matter the location or format — without moving it or duplicating it. This “data mesh” approach can then serve as a unifying data layer that helps organisations most effectively integrate their own data with generative AI.
Further, many public sector organisations have been finding it beneficial to use retrieval augmented generation (RAG) workflows to connect their proprietary data with generative AI applications. This allows them to securely reap the benefits of generative AI technology without compromising the privacy of sensitive data. It essentially serves as a “context layer” that sends only the most relevant data to a generative AI application.
When implementing this context layer between private data and public generative AI, a vector database is a critical determinant of success. A vector database can normalise and streamline data so that you can search it quickly, no matter what format it’s in. Because a vector database stores information as vectors, or numerical representations of data, it’s much easier to find and correlate data across traditionally incompatible formats, such as text, images, sensor data, and more. Having the ability to quickly find what you need from all data then enables you to send the right, context-rich information to generative AI applications, thereby increasing the accuracy and relevance of AI outputs.
Success story: How a university is using generative AI to increase students’ access to financial aid
As a longtime partner with the public sector, Elastic is working with many government, healthcare, and education organizations around the world to securely implement generative AI into their technology environments. The generative AI use cases for public good are myriad, but here’s just one example of what it looks like in action.
Imagine you’re a high school student applying to college. Your family is supportive but doesn’t have the financial means to pay for your tuition. You’ve heard about a few classmates who have gotten financial aid packages and scholarships, but you don’t know where to start. What opportunities are available to you? How do you apply? What schools can funding be used for?
One university set out to help potential students answer these questions, through the power of generative AI. Georgia State is a public university that serves 50,000+ students across the US state of Georgia and has been named the second most innovative university in the US.
The team set up a proof-of-concept for generative AI at the university, using the financial aid use case as its pilot. It’s an ideal use case for GenAI because there are so many disparate, publicly available resources on financial aid from multiple sources and formats. All this data is ingested into Elastic and turned into vectors (numerical values), which allows it to be searched semantically (or in a user’s natural language instead of through a specific keyword).
The financial aid process can be complicated as students try to understand whether they qualify for scholarships and grants. Students who need assistance but can't find funding may be less likely to complete their college degrees.
Jaroslav Klc, Director of Strategic Initiatives and Development for IT, Georgia State University
This in itself would be a benefit to students, but the team goes even further by setting up a retrieval augmented generation (RAG) workflow that adds critical context to the publicly available financial aid data via the university’s own proprietary data. This data supplies the generative AI model with contextual information as well as accompanying instructions to the GenAI model on how that information should be factored into the output. When students visit the university’s financial dashboard, they see personalized content reflecting their current status as well as information or alerts about external financial aid resources relevant to their status.
Considerations for public sector AI implementation
Take a privacy-first approach. It goes without saying that the data that public sector organizations are responsible for is often highly sensitive and subject to regulatory scrutiny. Using a platform like Elastic — with its role-based access controls — can provide a secure bridge between your organization’s own data and publicly available LLMs. Elastic’s power as a vector database is critical in the process, as it allows data to be searched and analyzed as numerical values with private information removed.
Make sure your data foundation is healthy. The quality of generative AI outputs is only as good as the data you give it. Make sure you have a way to see across your entire data landscape regardless of data format and location. That way, when a RAG workflow searches your organisational data for relevant context, it will have an accurate, holistic set of data to draw from.
- Modernise legacy IT for unified data and visibility. With limited budgets and disconnected tech tools, many government agencies find themselves piecing together legacy tools and systems that don’t speak to each other or offer a unified view of your environment. This is problematic when agencies begin using generative AI since the AI will be producing outputs based on incomplete, siloed, and outdated data.
- Save costs by avoiding fine-tuning. Although AI is poised to save public sector time and budget, behind the scenes, it can take a lot of effort to fine-tune a large language model (LLM) yourself because the job will never be done. There will always be more data to add. When you use RAG, however, the generative AI model will always pull from your most up-to-date, relevant data.
Learn more about Elastic’s approach to AI for the public sector.
Join us at Public Sector Summit!
Register to attend Elastic’s free Public Sector Summit in London on 25 September. You’ll hear how government leaders from 10 Downing Street, GCHQ, and more are leveraging democratised data as a strategic asset for AI insights, cybersecurity and more. You’ll also hear from Elastic’s own product experts on how our observability and security solutions are helping governments and education teams maximise value from huge amounts of data. Sign up now!
The release and timing of any features or functionality described in this post remain at Elastic's sole discretion. Any features or functionality not currently available may not be delivered on time or at all.
In this blog post, we may have used or referred to third party generative AI tools, which are owned and operated by their respective owners. Elastic does not have any control over the third party tools and we have no responsibility or liability for their content, operation or use, nor for any loss or damage that may arise from your use of such tools. Please exercise caution when using AI tools with personal, sensitive or confidential information. Any data you submit may be used for AI training or other purposes. There is no guarantee that information you provide will be kept secure or confidential. You should familiarize yourself with the privacy practices and terms of use of any generative AI tools prior to use.
Elastic, Elasticsearch, ESRE, Elasticsearch Relevance Engine and associated marks are trademarks, logos or registered trademarks of Elasticsearch N.V. in the United States and other countries. All other company and product names are trademarks, logos or registered trademarks of their respective owners.